In the competitive market of differentiated and specialty chemicals, Huntsman Polyurethanes focused on further digitization of the plants, starting with leveraging time-series data. A self-service analytics platform was selected to help the process, and asset experts contribute to their corporate operational goals. Next to the technology tools, the journey also was, and still is, about organizational and people aspects.
As operational data was kept in separated silos, this added an extra challenge to improve operational excellence by the use of advanced analytics. The lessons learned help new sites quickly adopt the new self-service analytics tool and benchmark site performance independent of the exact location where the original data is stored. The various use cases addressed with the analytics platform provide benefits in many areas. One of the most important is an increase of stable operations, also directly leading to a safer production site.
Business challenges
Within Huntsman, like any other process manufacturing company, operational data has been gathered for years. Typically, the engineers are using their many years of experience in combination with the data for their daily work. So, one might think they work data-driven. But in fact, this is experience-driven, as it is coloring the data with its own experience.
Figure 1: "Methods, management and mindset" capture a way of working, but mindset is the most critical—people having the mindset to use certain tools and wanting to make it a success. Source: TrendMiner
The journey within Huntsman actually is to come from experience-driven to data-driven. A common challenge is related to data skills: Have the process engineers talked with the data scientists? Where the engineers are looking at trends and interpreting them, today they have to talk with a data scientist. However, the data scientist thinks in algorithms and statistical models. So, one has to find a common ground for those disciplines to start talking to each other.
The other challenge is related to the way of working, captured in “methods, management and mindset” (Figure 1), where mindset is what it’s really about—people having the mindset to use certain tools and wanting to make it a success. That doesn’t mean that you have to forget the other two. A successful transformation addresses all three aspects. If you take out one of these aspects, you don’t have a successful transformation.
Self-service advanced analytics
To really use the sensor-generated time-series data, the solution is to create a so-called analytics engineer. You can empower engineers with advanced analytics or make data scientists more process-oriented, as long as you know where you want the person to operate, and that they know what they need to be able to do it and to talk to the various disciplines. Because companies already have the engineers who are used to looking at data trends from their historians, it’s easy to empower them with new plug-and-play tools.
A few years ago, our team at Huntsman Polyurethanes selected a self-service analytics tool to go from pure diagnostics (what has happened and why?) to something that tells you what’s behind this trend. By using self-service analytics, the engineers became much more effective in looking at trends, searching back in time and comparing good with bad situations, to come up with a solution in case of an operational problem.
The basis of the self-service advanced analytics platform is rapid-fire pattern recognition. The first step is to analyze the patterns, what do the patterns tell; look at the past, compare patterns and see good and bad behavior of the production process.
One can consider this 24-hour engineering support. A lot of engineers follow patterns, but they are not present 24/7 in your company. So, if you put their knowledge in a monitor that creates alerts via messages, email or dashboards, it actually provides a kind of 24/7 engineering support (Figure 2).
Figure 2: Many engineers can follow patterns, but they are not present 24/7. Putting their knowledge in a monitor that creates alerts via messages, email or dashboards can provide a kind of 24/7 engineering support.
The next step is to use the tool to monitor those patterns, followed by using the monitors to predict operational performance and create early warnings with messages (Figure 3). You can do that for operating conditions, and also for maintenance. Lastly, for continuous improvement, the self-service analytics tool can be used in each phase of the classic define-measure-analyze-improve-control (DMAIC) cycle used by engineers and in the six-sigma methodology to improve operational excellence.
Soft sensor on quality
In one use case, a Huntsman continuous isocyanate plant has been collecting process data for years and collecting offline-created lab analysis data daily, both stored in the historian. It was quickly discovered that combining the two types of data can be used to build soft sensors, predicting what the quality is going to be. This soft sensor is used to make micro-adjustments to process setpoints to reduce the frequency of off-spec production. A huge added value became quickly clear. Because some analysis can only be done during the week, while production also runs over the weekend, the system can already predict whether a certain parameter is going to be off-spec during the weekend. Early warnings via the monitors can tell the operators not to load the truck, preventing off-spec material from going to the customer. On the other hand, when the operator isn’t sure and they load the truck, the laboratory can confirm that the quality is ok. This has a big positive impact on lead time as well as on quality control.
Fingerprinting batch processes
Probably a lot of people are checking profiles of batches in the classic way. In this second use case, we were doing that in MS Excel, which was very laborious and required a lot of expertise. Today, this is done by creating fingerprints with the self-service analytics tool, telling whether a batch is produced in-spec or not.
Figure 3: Anticipating, predicting and identifying changes as soon as possible are three ways that early warnings from statistical analytics provide opportunities to control operational performance. Source: TrendMiner
In a specific batch process, very distinct pressure and temperature profiles were required to consistently create high-quality material. Time series patterns from known good batches were grouped and saved as a “fingerprint.” The golden batch fingerprint is then used as a real-time monitor to continuously check the process for deviations. Subtle disturbances that would be difficult to capture in a numerical model are quickly identified using the fingerprint. So, there is no need to check afterward if there have been some abnormalities. The monitors give early warnings during a batch process in case something is going wrong, allowing the operators to take appropriate action in time. The new way of batch analysis led to a reduction in off-spec batches and increased quality of the product.
Recently, a young engineer—think about the new way people are working—who works daily with the analytics platform had to do this work without this tool available. The old-fashioned way to execute this work turned out to be much more laborious. Afterward, the engineer emphasized the need to have this modern platform always available.
Quality improvement using DMAIC
In a third case, a Huntsman advanced materials site in the United States experienced that many batches run in a wiped-film evaporator exceeded the specification limit of unreacted feedstock, resulting in off-spec products. They were observing a multiyear drift in quality as measured by the QA lab. It was suspected that this was due to a change in testing methods, however, the frequency of off-spec production demanded a resolution.
A complete six-sigma DMAIC analysis was done with various capabilities of the self-service analytics platform, such as vale-based searches, layer compare, statistical comparison tables, recommendation engine, filtering and the use of scatterplots. Multiple differences in the plant between on-spec and off-spec production campaigns were revealed. Through area search in scatterplots, it is quickly clear if performance is in or out of the best operating zone. The differences were enough to convince subject matter experts that there were long-term changes occurring in the process. Through further investigation the root cause was identified and resolved, and immediate quality improvements were confirmed days later.
The result was that the problem analysis can be done much quicker, on a much larger data set identifying subtle operating differences, and more tests can be done. A side result was increased trust in the lab data. With the related test data from the laboratory, everybody can now quickly see if the lab data can be trusted or not.
Benefits, results and learnings
Besides employee efficiency and truly making data-driven decisions, the use of self-service analytics helped break down organizational silos. Another big value of self-service analytics lies in easily and quickly built soft sensors. They help to monitor the operational performance, but can also help at startup of the production line. Even if certain sensors are not working properly, a soft sensor can be created quickly using other parameters to help control a safe start-up process.
Along with product quality improvements, improved capacity without capital investments, and the creation of the data-driven “24-hour engineering support,” we were able to improve process and asset reliability. Higher reliability leads to a more stable, and therefore a safer, plant.
About the author:
Jasper Rutten, advanced analytics manager, Huntsman, can be reached at [email protected].
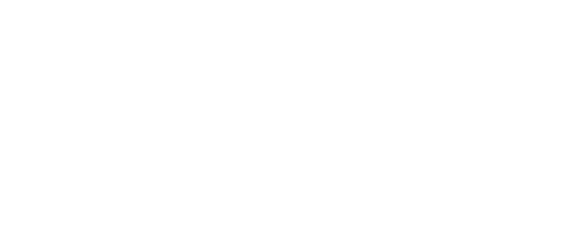
Leaders relevant to this article: