Operational performance criteria and the future of multivariable control
Loop tuning has always been a challenging part of process control. In principle, its methods are well-known, but in practice, it often involves rework, ad hoc adjustment and “detuning." While the entire process control community (and not a few operations managers) wish loop tuning behaved like a reliable one-time activity, it often feels more like recurring maintenance.
In recent years, multivariable control has emerged with the same behavior. Plant step-testing and modeling were conceived as one-time activities, but industry practice today routinely includes re-modeling, model maintenance, and model performance monitoring.
The two activities (tuning and modeling) are fundamentally the same – ascertaining actual process gains* in order to derive controller settings – so in retrospect it is more edifying than surprising that they have encountered the same challenges. Indeed, the two difficulties share the same root causes.
One root cause is that process gains change, not just with long-term affects such as heat-exchanger fouling and catalyst deactivation, but also with short-term factors such as feedstock quality, feed rates, ambient conditions, equipment performance, product specifications, severity, equipment selection, and many more. In short, the process disturbances we seek to control often alter the very tuning parameters (or models) we employ to control them. It basically breaks loop-tuning and model-based control theories when process gains change from the tested values. It is this root cause that industry has best understood and most tried to remedy with better modeling and tuning tools, albeit (in the author’s view) with an insufficient sense of the dynamic nature of the problem.
Figure 1. Industry’s de facto control performance criteria is error-minimization, but industrial process operation (and other high-consequence activities, such as piloting passenger jets) normally place higher emphasis on preserving process stability and operational pre-caution, which are represented by the 1st order and ramp lines.
But a second equally fundamental root cause has also been at play that has largely avoided detection, even as it has undermined process control performance for over half a century. From Ziegler-Nichols to model-predictive control (MPC), industry’s de facto control performance criterion has been error-minimization. (“The objective of any control scheme is to minimize or eliminate error,” 1 is a typical control literature statement.) But experience has now shown this to be an inappropriate performance goal for many industrial process control applications, especially for high-level control, such as MPC. Figure 1 depicts that error-minimization criteria is fundamentally aggressive and results in behavior such as overshoot and oscillation, whereas actual industrial process operation normally places greater emphasis on carefully preserving process stability, with an eye to process safety, reliability and mitigating risk. In short, when it comes to control loop performance, whether single-loop or multivariable, operational pre-caution takes priority over error-minimization.The interplay of multiple root causes (there are more2) has contributed to their persistent obscurity, but circumstances today serve to reveal the big picture. One circumstance is the telling parallels between the improvised rework practices of both tuning and modeling, which point us to look for common root causes. Another is that modern computer-based tools have not resolved tuning and modeling issues once and for all, thereby revealing that the accuracy of data analysis and modeling tools is not the limitation. Another clue is the prevalence of single-loop “detuning” and MPC “degraded” performance, which tells us that traditional tuning methods generally result in operationally over-aggressive performance. Another telling observation is that detuning and degradation often occur even where models are durable, thereby confirming the primacy of operational performance criteria over control performance criteria.
This perspective stands much of process control on its head: Industry’s traditional control performance criteria, and the underlying basis of essentially all tuning and model-based control methods, turns out to disregard a normally higher-priority (operational) performance criteria. Moreover, the methods themselves are found to have a fundamental vulnerability (process gains that change dynamically).
Embracing this perspective gives pause, because it challenges long-held paradigms (that identifying gain is the key to success) and initially would appear to render tuning and modeling all the more intractable, rather than moving industry towards a solution. But pursuing this perspective indeed has the virtue of revealing new solutions that not only address the root causes, but also promise to be less complicated, more robust, and even to transcend (bypass) some of the more taxing aspects of conventional practice, especially detailed tuning and modeling, which become largely unnecessary where process gains change or strict error-minimization is not the main priority.
- Pre-selected MV move rates based on operating experience and procedures.
- A ramp or first-order approach to targets and constraints (not "quarter amplitude decay")
- Little or no overshoot, for both the MV and the CV
Rate-based control (RBC) uses approximate process response time and ongoing rate of change of the controlled variable to taper (reduce and halt) pre-selected move rates in a predictive manner so that the controlled variable ultimately settles exactly on the target value without overshoot or cycling. This mechanism is depicted in Figure 3 and derives from the basic mathematics and dynamics of first order systems.
Figure 3. Rate-based control (RBC) uses process response time and controlled variable rate-of-change to taper (reduce or halt) pre-selected manipulated variable moves in a predictive manner that results in the CV settling on the target value without overshoot, cycling or other operationally undesirable behavior. Moves are tapered when the predicted value equals or exceeds the constraint or target value.
That’s one pleasant surprise – that achieving operational performance takes only a modicum of control engineering savvy, not rocket science. But what about the other root cause, changing process gains? It also turns out that RBC is inherently adaptive to changes in process gain. For example, if process gain doubles (for whatever reason), then the process response will double and the RBC moves will be tapered correspondingly sooner, again resulting in the controlled variable landing right on target. Moreover, the same holds true for changes in the predefined move rate, which brings further practical advantages, because it means that move rates can be adjusted to achieve desired operational performance without impacting control performance. And, incidentally, thereby giving industry perhaps its first truly inherently adaptive control algorithm – a pleasant surprise indeed!If this method sounds vaguely familiar and intuitive, it may be because it largely mimics (automates) time-honored (pre-computer) manual operating practices, which (perhaps it has been overlooked in the focus on gains and computerization) by necessity took operational performance criteria and dynamically changing process gains into account. Most industrial processes have essentially always been managed and operated this way, aided (or not) by automation.
RBC has obvious applicability to high level control, where it is fundamentally important to move setpoints and outputs at deliberate rates that allow the base-layer controls to keep up and maintain process stability. RBC also has potential base-layer single-loop applicability (where the manipulated variable is the output, the controlled variable is the process variable, and the target is the setpoint), especially for critical loops where stable operational performance is more important than large fast proportional or derivative control actions.
In industry, “detuning” is as common as “tuning”, and MPC “degraded performance” affects the majority of installed applications3. Somewhat sadly, this has become accepted as the norm, rather than the bane, of process control. Understanding the root causes and designing improved solutions, such as those outlined here, is critical to move process control beyond the troublesome performance plateau where it has resided for decades, and to re-establish enthusiasm for the power of process automation to operate processes more safely, reliably and economically.
Footnotes
Process “gain” is the familiar usage, but technically it comprises the entire process response, including the interim dynamic response and the final steady-state gain.
References
- Instrumentation and Control, Process Control Fundamentals, www.PAControl.com, 2006
- Multivariable control performance: the case for model-less, by A. G. Kern, InTech, July-August, 2014 https://www.isa.org/intech/20140802/
- Successful APC: Design and Maintain for Long-Term Benefits, by Gary Jubien and John Mcllwain, ISA EXPO 2009.
The following is a comment from Sigifredo Nino, P. Eng:
[sidebar id =4]
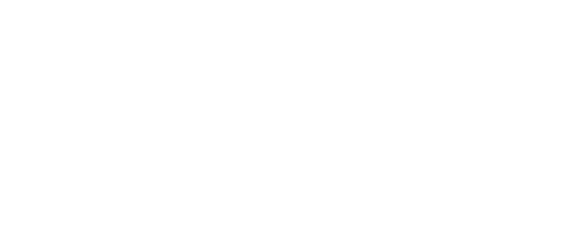
Leaders relevant to this article: