Combine todayās seemingly limitless computing resources with cutting-edge, deep-learning algorithms and what do you get? An industry-wide fascinationābordering on obsessionāwith the promise of data analytics to unearth solutions to unknown problems and transform the performance of plant operations.Ā
Peter Zornio stresses that while data analytics certainly have their place, itās also important to remember that in many decision-making scenarios across industrial manufacturing the best analysis isnāt based on statisticsāno matter how high-powered. Instead, a demonstrated understanding of first principlesāof how things work as well as of chemistry, physics, fluid and thermodynamicsācontinues to play an essential role.
Zornio is chief technology officer for Emersonās Automation Solutions business, and has guided development of what the company calls its āoperational analyticsā portfolio, to distinguish these solutions from the broad swath of general-purpose analysis tools available in the marketplace. Control caught up with Zornio at Septemberās Emerson Global Users Exchange in Nashville, Tennessee, to learn more about the companyās deepening portfolio of operational analytics developed for the process, hybrid and discrete industries.
Chief Technology Officer, Automation Solutions, Emerson
Q: Analytics has been a major theme of this weekās conference, but I think some people in the automation space are a bit intimidated by the concept, thinking that all analytics require some sort of data science degree. But at the operational level, analytics donāt have to be all that complicated. Can you explain where analytics fit within the traditional paradigm of sensors, controllers and actuators of industrial automation?
A: First of all, I would say that control and automation people should not be afraid of analytics, because they've been using them for a long time. We just didn't necessarily always call them that. The broad category of analyticsājust as the name impliesāis doing analysis, modeling or any other kind of processing of data to derive what a future condition might be based on current conditions. Also, analytics seek to establish causal relationships and to perhaps to build an online model that looks at current conditions and tells you what actions to takeāor maybe even takes actions itselfāto provide better performance, prevent a failure or otherwise do something to make the world better.
In our case, āanalyticsā could be as simple as what weāve done with PID loops for 60 years now. A PID loop is fundamentally a very simple model. We look at the data and we take action.
What weāve talked about at Emerson Exchange is that there are lot of different kinds of analytics that we've always used in our industry. Plants themselves have been designed with what we call principle-based analytics, for when we know the actual interrelationships or the chemical, physical or thermodynamic rules of how things work together. We sometimes call those mechanistic models, and that's how plants are designed: we know the chemistry, the physics of how things work.
We also have for a long time used in our industry what is called FMEA, or failure mode and effects analysis models, where we know first-principle relationships like ācars need gas to run,ā right? So if your car's not running, check the gas before you actually start looking at databases. We've used those kinds of rule-based analytics for a long time.
What all the hype has been about lately is data-driven analytics, this idea that just by looking at the data itself you can establish these relationships; you can build a model without actually knowing anything about those first principles of how they interrelate. We used neural networks some 20 years ago, and embedded that capability in our control systems.
But lately, because of tremendous increases in computing horsepower together with advances in machine learning, the ability to recognize patterns and create data-driven models has become quite good. We've learned how to build models just by training them with extensive data sets, by letting these algorithms look through a lot of data.
Analytics used for decision-making in the realm of process, hybrid and discrete operations fall into two major groups, those based on analysis of patterns in data and those based on an understanding of how things work.
Q: What is an example of a problem that is best solved through principle-based analytics versus a more complex approach?
A: One example would be if you're trying to diagnose what's going on with a heat exchanger. Every second-year chemical engineering student knows how to do a mass and energy balance around a heat exchanger, and knows how to figure out the heat transfer coefficient by looking at the inlet and outlet temperatures. From that, they can figure out if itās behaving in the way it was designed or if itās fouling. You don't need to look at years of data from that heat exchanger.
When you need something more than first principles is when the system gets more complex, or you have a system of systems that are interacting.
In that case, it can become super complicated to put together all the rulesāeven if maybe you might know themāto define what that whole system looks like or how it behaves. Thatās when it becomes easier to go to a data-driven model.
Q: The terms big data and cloud are often attached to the term analyticsābut clearly not all analytics should run in the cloud. Can you talk a little bit about philosophy in terms of where people should be thinking about deploying analytics in the operational technology space?
View the live coverage of Emerson Global Users Exchange 2019
A: We use the term āoperational analyticsā to differentiate them from the sorts of analytics people might be running at the enterprise level, where they're looking at retail behavior of customers or maybe the best procedures for hiring employees. You can apply analytics to really just about any kind of problem or any kind of situation. So we talk about operational analytics as solving process, production or operations issues to improve plant performance.
Back to that heat exchanger we discussed earlier, we know how it works, and its data lives on-premise, at the site. Cloud horsepower isnāt needed to run that model. And, in fact, the person who needs to take action based on what that model tells you is someone at the plant. So, why not have someone at the plant level be in charge of what's happening with the analytics as well as being accountable for taking corrective action when the analytics tells them that something needs to be done? On the other hand, cloud-based analytics are perfectly appropriate when you want to compare that same heat exchangerās performance across a fleet of similar equipment perhaps deployed in different locations.
The Emerson portfolio has long included such operational analytics based on first principles and domain expertise, and our recent acquisitions of iSolutions and KNet have significantly bolstered our ability to deliver data management services and leading-edge data analytics technologiesāwhether on-premise or in the cloud. We also continue to develop new, easy-to-implement sensor technologies for variables such as corrosion, location and vision, and can today bring to bear, on behalf of our customers, an increasingly comprehensive, top-to-bottom suite of operational analytics solutions.
Editor's Note:Ā This post was sponsored by Emerson Automation Solutions. To learn more about Emerson can help with your operational analytics, visit Emerson.com.
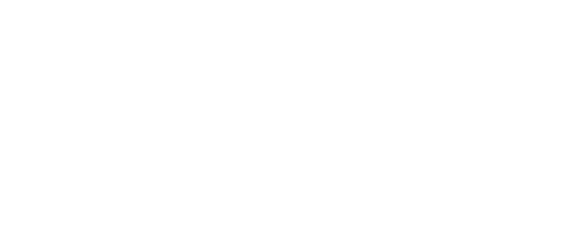
Leaders relevant to this article: