Solutions spotlight: Operational analytics go beyond data science
Control publisher Keith Larson guest hosts this Solutions Spotlight edition. In this podcast, Keith speaks with Peter Zornio, chief technology officer for Emerson's Automation Solutions business to talk operational analytics.
Transcript
Keith Larson: Hi, this is Keith Larson, publisher of Control magazine and controlglobal.com. Welcome to this Solution Spotlight edition of the Control Amplified podcast. Today, I'm on location at the 2019 Emerson Global users Exchange in Nashville, Tennessee, and I'm joined by Peters Zornio, chief technology officer for Emerson's Automation Solutions business to talk operational analytics. Welcome, Peter! A pleasure to have you here.
Analytics has certainly been a major theme of this week’s conference, and I think some people in our space are a bit intimidated by the concept, thinking that all analytics require some sort of data science degree. But at the operational level, analytics don’t have to be all that complicated. Can you explain where analytics fit within the traditional paradigm of sensors, controllers and actuators of industrial automation?
Peter Zornio: First of all, I would say that control and automation people should not be afraid of analytics, because they've been using them for a long time. We just didn't necessarily always call them that. The broad category of analytics—just as the name implies—is doing analysis or modeling or any other kind of processing of data to try to derive what a future condition might be based on looking at today's conditions. Also, to establish causal relationships and to perhaps to build an online model that looks at current conditions and tells you what actions to take—or maybe even take actions itself—to provide better performance, prevent a failure or otherwise do something to make the world better.
In our case, “analytics” could be as simple as what we’ve done with PID loops now for 60 years now. A PID loop is fundamentally a very simple model of what we think the process looks like in terms of its control parameters. We look at the data and we take an action.
What we’ve talked about here at Emerson Exchange this week is that there are lot of different kinds of analytics that we've always used in our industry. Plants themselves have been designed with what we call principle-based analytics, for when we know the actual interrelationships or the chemical, physical or thermodynamic rules of how things work together. We sometimes call those mechanistic models, and that's how plants are designed: we know the chemistry, the physics of how things work.
We also have for a long time used in our industry what is called FMEA, or failure mode and effects analysis models, where we know first-principle relationships like “cars need gas to run,” right? So if your car's not running, check the gas before you actually start looking at databases. If you have a gas gauge, you don't need a neural network to tell you you're out of gas. So those kinds of analytics have been used for a long time in our industry. What all the hype has been about lately is data-driven analytics, this idea that just by looking at the data itself you can establish these relationships; you can build a model without actually knowing anything about those first principles of how they interrelate.
We used neural networks some 20 years ago, and embedded that capability in our control systems. But lately, because of tremendous increase in computing horsepower together with advances in machine learning and deep learning, the ability to recognize patterns and create data-driven models has become quite good. We've learned how to build models just by training them with extensive data sets, by letting these algorithms look through a lot of data.
KL: Can you give us an example of a problem that is best solved through principle-based analytics
versus a more complex approach?
PZ: One example would be if you're trying to diagnose what's going on with a heat exchanger. Every second year chemical engineering student knows how to do a mass and energy balance around a heat exchanger, and knows how to figure out the heat transfer coefficient by looking at the inlet and outlet temperatures. From that, they can figure out if it’s behaving in the way it was designed or if it’s fouling. You don't need to look at years of data from that heat exchanger: you know exactly how it should behave based on the heat-transfer equation. So, go ahead and make that model based on those first principles and use it to troubleshoot what's going on with the heat exchanger.
KL: So it's math but it's not statistics.
PZ: Correct. That's a great way to put it out. It’s math; it's thermodynamics, it’s the physics and chemistry of the system. When you need something more than first principles is when the system gets more complex, or you have a system of systems that are interacting. In that case, it can become super complex to put together all the rules—even if maybe you might know them—to define what that whole system looks like or how it behaves. That’s when it becomes easier to go to a data-driven model.
Training a car how to drive itself is a great example. If you tried to express in first-principle terms all the things that could occur—all the potential scenarios—that would very hard. So, instead you start with a lot of first principles, like when you step on the brake the car is going to slow down, or when you step on the gas the car will speed up. Those models all start with some of those basic principles, but then are trained with lots and lots of real world data and experiences of what driving is really like. They learn how to do many of the more subtle and advanced things that humans are typically very good at.
KL: The terms big data and cloud are often attached to the term analytics—but clearly not all analytics should run in the cloud. Can you talk a little bit about philosophy in terms of where people should be thinking about deploying analytics in the operational technology space?
PZ: We use the term “operational analytics” to differentiate them from the sorts of analytics people might be running at the enterprise level, where they're looking at retail behavior of customers or maybe the best procedures for hiring employees. You can apply analytics to really just about any kind of problem or any kind of situation. So we talk about operational analytics as solving those process, production or plant operations issues right to improve the performance of a plant.
Back to that heat exchanger we discussed earlier. We know how it works, and its data lives on-premise, at the site. Cloud horsepower isn’t to run that model. And, in fact, the person that needs to take action based on what that model tells you is someone at the plant. So, why not have someone at the plant level be in charge of what's happening with the analyticS as well as being accountable for taking corrective action when the analytics tells him that something needs to be done.
KL: Cloud horsepower has increased dramatically of late, but the edge is now much more powerful as well. A lot more can be done locally.
PZ: Very true. Edge is another interesting term that has come to mean a lot of different things to different people. I personally believe that edge was a term invented by the cloud guys because they didn't know what to call all that stuff that happens down in the plant. I think in our industry, when the term edge is used it basically means that the calculation is happening at the plant level, very close to where the data is generated—maybe in a device mounted right next to the machine. But it also could just mean that the calculation is happening in a system that is on premise at the plant. It could be a very low horsepower counter device mounted at the side of the machine, or actually a big server that just happens to be on premise running a much more substantial kind of model.
Back to the example of the self-driving car, think about the analytics that are going to talk to the sensors and figure out if that object in the road is a beach ball or a boy. You want those running in the car. But when it comes time to look at all the sets of cars that have had to make that distinction, and using those data sets to improve the algorithm that's running in all of the cars, that's a perfect job for the cloud.
KL: I understand Emerson has made some investments recently to help its customers solve problems that might require some higher-level data analytics capabilities. Can you talk in a bit more detail about the KNet acquisition and how it fits into the existing Emerson portfolio?
PZ: We’ve had a long history with what I’d call field-level analytics: the original Plantweb that Emerson launched in 1996 was all about running diagnostics and analytics in our own devices, to detect things like entrained air in a Coriolis meter. We've also done things like monitoring for vibration on rotating equipment. Over the past decade we’ve also introduced other packaged analytics for a range of asset classes like pumps and heat exchangers.
What we didn't have was kind of that broad-scale, machine-learning platform—featuring all of the latest and greatest techniques—that either we could use to develop applications or that our customers could use themselves to address bigger problems. We acquired the KNet technology to fill that slot in our portfolio.
Now we feel we've got it covered from top to bottom: embedded diagnostics that are in the devices themselves; packaged applications for single, simpler assets that are frequently first principles based; all the way up to the KNet platform where we can tackle bigger problems with data-driven analytics.
KL: Obviously sensors are at the heart of both kinds of analytics whether its data analytics or first-principles analytics. What are the new frontiers of sensor technology that you're exploring or expect to be more broadly deployed in the next few years?
PZ: For a dozen years now we've had this strategy that we call pervasive sensing which is really about a whole new portfolio of sensors to be applied to areas just outside of the traditional process measurement domain. Broadly speaking, we were looking for sensors that, number one, would be much simpler to install. We’re often talking about brownfield sites, and nobody wants to have to go cut into a pipe for weld. So, making these new sensors non-intrusive, making them simply clamp on, and making them easy to install in a process was a primary criterion. Number two was making it easy for them to communicate, which meant not having to run wires. Wireless technology has played a huge role in enabling the deployment of new sensor technologies. Finally, there are new sensor types, including those for measuring corrosion and acoustic sensors for detecting leaking steam traps or the activation of pressure relief valves. We had vibration sensors before, but are now able to deploy them more easily. Location awareness is one that we just introduced and already is proving to be very popular.
Looking further ahead, we'll continue to expand the portfolio that we have with existing wireless technology, but we’re always looking at what a next-generation wireless technology might be. Vision, too, is of interest, and not just for discrete manufacturing applications. In the process world, we see people thinking of using vision to identify whether people have the right equipment, to make sure that people aren’t where they’re not supposed to be, or even using vision to physical recognize where you are in a plant to provide location context.
KL: Many operational analytics opportunities are of course in brownfield facilities where there are many disparate sources of data. What steps has Emerson taken to ease the often complex task of bringing those sources of operational and enterprise data together?
PZ: That is a big challenge, and from my observation kind of what happened is that first we had a huge wave of excitement about analytics and machine learning and all this new stuff. Everybody ran out to work on it and then all of a sudden realized they didn’t have the data gathered together in a consistent context where it could be used and digested by the analytics. A lot of our customers have found out that there's a lot of hard work to be done still in their data-integration strategies and how they bring the data together. This need, amongst other factors, drove us to acquire another company called iSolutions, out of Canada. They specialize in data integration projects, helping customers to develop a strategy and a sustainable data infrastructure to enable these kinds of analytics applications.
KL: That certainly makes a lot of sense, and it sounds like what pieces you didn't have already you've taken some good steps to bring together. I appreciate you taking the time to update us and our listeners on the latest work you've been doing to bring your end users along and really put operational analytics to work.
I'm Keith Larson and you've been listening to the Control Amplified podcast. Thanks for joining us. If you've enjoyed this episode you can subscribe to future episodes at the iTunes Store and on Google Play. Plus you can find the full archive of past episodes at controlglobal.com. Signing off until next time.
Latest from Data Acquisition
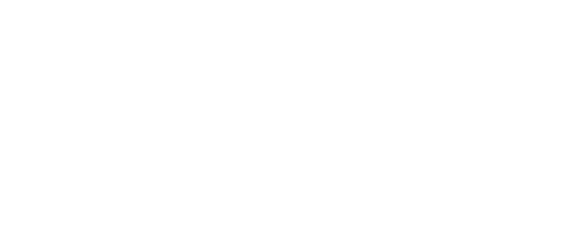
Leaders relevant to this article: