XPS achieves fast ROI with analytics solution
As an expert in process development and operational support in the mining, mineral processing and smelting/refining operations, XPS (Expert Process Solutions) has long been an innovator in the metals and mining industry. Over many years of operation, we've developed advanced modeling and analytics to deliver practical processing solutions for improving efficiency and operational excellence across a wide range of clients and partners.
One of the key features of a good analytics program is turning data into action to immediately save time and resources. Today, systems leveraging artificial intelligence (AI) and machine learning (ML) techniques can process and analyze multivariate data that wasnāt possible before, providing fast answers to complex production problems and delivering those solutions straight to operations and maintenance personnel with actionable guidance. However, these powerful analytics tools require a strong technology stack. Properly developing a technology stack is a critical processāone that does not occur by chance.
The XPS process control and analytics team had great success in developing an advanced analytics program incorporating AI and ML to deliver fast returns, but it wasn't designed in a vacuum. The team identified and never lost sight of three key areas of focus: collection capability, robustness of analytics and the capacity for fast, demonstrable return on investment (ROI). Any organization using these same criteria should be able to identify the analytics technologies and strategies best matching its own dynamic environment.
Engineering the system selection process
The XPS analytics team knew it needed a clear and defined process for choosing the technologies to drive its analytics program. Initially, we explored the option of designing our own system in-house from scratch. That idea was quickly dismissed, however, as such a system would likely have been complex and difficult to maintain, generating a high total cost of ownership (TCO) over its lifecycle.
We instead opted to implement an existing analytics software package as a foundation to help the organization move its complex models online. While it was possibleāand even temptingāto just select one of the many analytics systems available on the market and get started, the XPS team wanted to ensure its technology core would be able to handle the complex models it uses today, while building a foundation for future development capable of delivering fast returns. To accomplish this goal, a select group of team members intensively benchmarked 10 analytics packages from different vendors to see which best fit the XPS environment and the unique needs of its customers in the metals and mining industry.
The process of benchmarking so many systems was time consuming, but it provided benefits that quickly offset that investment. Team members could see the advantages and limitations of each solution, not only as a list of software features, but as they actually applied to XPS clientsā production. Running side-by-side with existing systems, the team could see where each solution succeeded or fell short, and how quickly each one would drive key changes.
Greater connectivity provides better data access
XPS must work with control systems and applications from a variety of vendors to effectively deliver its services. As various solutions are added to tackle different use cases, such an environment can quickly develop into a web of complex, custom-engineered interfaces between disparate systems. As complexity increases, operation, troubleshooting and maintenance can become cumbersome, reducing or eliminating the efficiency gains offered by an analytics solution.
Figure 2: Maintenance and operators thought the filtration problems occurred when the level was within a certain range, but many normal filtration times occurred during this time as well. The team knew another issue needed identification.
To avoid adding complexity to the XPS architecture, one of the most critical benchmarks for the new analytics system was vendor neutrality. The team specifically searched for a platform able to integrate with a wide variety of control systems, historians and other information sources out of the box without custom engineering. Easy integration would allow for simpler installation and configuration, and give XPS the flexibility necessary to continue adding systems as required in the future without committing to one vendor.
Improved connectivity also provided expanded access to essential information. A wider range of systems connected to the analytics platform meant a wider range of data driving critical decision-making models, providing a foundation for more robust and accurate results.
The analytics team filtered out any solutions not meeting XPSā interoperability standards. The team wanted easy integration to enable XPS personnel to quickly bring together the data they need all in one place. Instead of hopping between stations or changing screens to collect data, the team wanted analysts and operators using the analytics platform to find value-added information in a single screen, helping them make and implement faster, better decisions.
Robust analytics enable flexibility
To determine the robustness of systems, the analytics team first evaluated the strength of the rule logic, AI and ML, and automated failure mode and effects analysis (FMEA) of each system. We quickly discovered that most systems provided all these features, but they couldnāt all be easily incorporated with the work XPS analysts and engineers had already created.
As the team narrowed its options, we focused on ways the systems enabled XPS engineers to modify, incorporate, and test existing analytics solutions. XPS engineers had already leveraged their expertise to design many analytical tools and models that would continue to be used after a new solution was in place. As a result, the right system would not only deliver new models and a strong suite of tools for development, but also provide a sandbox environment in which the team could leverage its own models, ideas, intellectual property and expertise.
Figure 3: The team pulled more data and used a Plantweb Optics Analytics multivariate analysis tool, principal component analysis, to cluster the data and identify distinct operating modes.
We found that the best systems were the ones that enabled engineers to build or use existing algorithms or rules offline, and test them before deploying to an online system. Truly robust systems avoided the need for the team to start from scratch by combining unique XPS knowledge with key out-of-the-box capabilities, all without sacrificing engineering best practices.
The team also ran old models side-by-side with new models to evaluate performance. With the data available from side-by-side analysis, XPS ensured the new models performed as expected and delivered ample value for the resources invested.
In the end, the XPS team selected Emersonās Plantweb Optics Analytics because its ability to meet or exceed benchmarking expectations demonstrated the potential to drive better analytics performance across the lifecycle of our customersā operations. But the most essential measure of success for the new XPS analytics platform was its ability to deliver ROI. The analytics team developed a prioritized list of high-value applications at two sites comprising both a mill and a smelter. The team then developed performance expectations and thresholds. With concrete, targeted goals in hand, the software was thoroughly tested to ensure it not only performed as expected but would also deliver fast ROI over its lifecycle.
Analytics in action
One targeted application projectāidentifying root cause of complex production issuesāwas completed well before the team expected. XPS had recently been made aware of a pumping issue that was causing cycle times to be 10 times longer than normal in a batch filtration process (Figure 1).
Observations from operations personnel indicated that this seemed to occur when the level in the agitated feed tank was within a certain range. The initial XPS investigation of historical data confirmed that, for the most part, the extended cycle times did occur when the tank level was within the particular range. However, the investigation also showed that extended cycle times also occurred outside of the level range, and furthermore, normal cycle times occurred within the particular level range with a greater frequency than the extended cycle times. This was a clear indication that factors other than the tank level alone were contributing to the observed behavior, requiring a multivariate analysis (Figure 2).
Figure 4: With the clustered data, the team built a decision tree to identify the root cause of the problemāthe spare thickenerāvery quickly.
During a software training session, the team decided to use the pumping issue as a trial. Once a dataset was collected and organized, the team was able to identify in less than an hour that slurry density was the other contributing factor to the observed behavior using Optics Analyticsā multivariate analysis capabilities. At the time, the thickener that normally fed the agitated tank was out for extended maintenance, and a spare thickener was being used in its place. The underflow density controls on the spare thickener were not as effective and allowed greater variability in the slurry density than when operating with the normal thickener (Figure 3).
The XPS team was able to quickly analyze a second data set to confirm that extended cycle times occurred much less frequently when operating with the normal thickener. They also identified a maintenance need for one pump on the normal thickener. Plantweb Optics Analytics allowed XPS to identify the real root cause of the problem, and avoided time and resources being spent addressing only the symptoms (Figure 4).
The analytics team has also successfully designed new models and digital twins used for the control of metals and mining assets such as furnaces, converters and reactors. The models were built entirely within the new analytics software, taking advantage of the sandbox-style flexibility to incorporate the teamās expertise in designing thermodynamic models, and even combining some pieces of AI.
The team expanded the range of available data by connecting the analytics platform to all data sources required for the models. Flexibility of the system allowed them to pull data from laboratory information management systems, the control system, the historian and more, and merge it all into the models.
Proceeding with a plan supports successful outcomes
Implementing an analytics program can be overwhelming for an organization beginning its digital transformation journey. Many critical factors will depend on the unique dynamics of the organization, but there are a few commonalities providing value across all organizations.
Collecting data from a wide variety of sources helps unite disparate systems today, and leaves your organization more choices for future expansion. In addition, a robust, sandbox-style analytics platform provides the flexibility needed to allow you to leverage the work and knowledge you already haveāavoiding the need to start everything from scratch.
Actively pursuing these goals can help you meet the benchmarks set at the beginning of the process, all of which combine to generate faster ROI, and position your organization to successfully expand analytics to meet the challenges of next-generation manufacturing.
Behind the byline
A principal engineer at XPS Expert Process Solutions, Phil Nelson has over 20 years of experience in a range of process control projects in base metal mills, smelters, and refineries both inside and outside Glencore. Projects have ranged across basic controls, specialty controls including furnace power, loop monitoring, and other analytics and machine learning applications. Implementation platforms include PLCs, DCSs, data historians, specialty software platforms and other IT systems.
Nicolas Lazare, manager of process control at XPS Expert Process Solutions, has 18 years of experience in automation, control, instrumentation and metrology for mines, mills, smelters, metal refineries, utilities and power stations. His experience in operations was leading teams in charge of engineering, commissioning and maintenance related to process control, instrumentation and metrology for SociƩtƩ Le Nickel-SLN and Koniambo Nickel. Since joining XPS Expert Process Solutions in 2017, he's provided expert services to mining and metals companies worldwide. Projects have ranged across instrumentation, automation, functional safety, advanced control and analytics, machine learning and digital transformation.
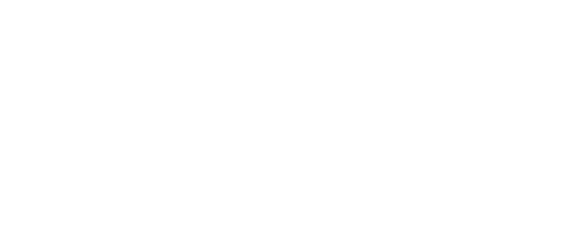
Leaders relevant to this article: