Motor gasoline is blended at the refinery to specifications that include octane rating, typically grades of 87 and 93 octane; at the pump these may be further blended to produce an intermediate grade such as 89 octane. An engineer I met was especially proud of how well octane number was controlled at his refinery—but he always filled his car at a competitor's station, although the price per gallon was the same. His rationale was that the competitor's control over octane number was more variable, but still had to meet the minimum standard of 87 for regular grade—hence its average octane rating was higher by that variability. The competitor's refinery was giving away octane, and he was willing to accept it. The analysis below can be used to describe the cost of giving away any valuable ingredient, or any operating cost associated with the variability of control.
Safe-Side Variations
The cost to the refinery in excess octane giveaway is a direct function of its variability, with two possibilities shown in the step-load response curves of Fig. 1. The upset could either drive the controlled variable in the "safe"direction shown in the black curve, or the "unsafe"direction, shown in red. Here, "safe"is meant to infer that the controlled variable is driven toward the favorable side of the specification limit, in this case above the 87-octane specification. Safe-side variations do not require any action either by the operator or any automatic response, because product specifications are not thereby violated. (Although there is a slight overshoot of setpoint on the return trajectory and therefore a momentary violation, any downstream capacity at all would absorb this brief transient, and it would not appear in the final product.) Safe-side variations simply result in an economic loss that is quantifiable.
Fig. 1. Octane giveaway is calculated from Integrated Error above specification limit.
If the setpoint in Fig. 1 is also the specification limit, and the measured octane rating rises due to the upset before returning to setpoint, the octane giveaway is related to the integrated error of the controlled variable from setpoint. Integrating this deviation or error e between controlled octane and its setpoint over time t gives the Integrated Error IE in terms of octane-minutes. Subsequent multiplication by the current product flow rate in gal/min yields octane-gal given away during that interval. Multiplying that result by the cost difference in the dollars/gallons/octane number converts the integrated error into dollars lost.
The cost function may not be linear. For example, the price difference at the pump between 87 and 89 octane, and between 89 and 93 octane may both be $0.10 per gallon, which probably reflects market forces rather than production cost. Nevertheless, small variations in the function around a given specification can be assumed to be linear, allowing IE to be a useful criterion in evaluating the cost of poor control.
When using PI or PID control over any variable, integrated error is directly related to the size of the disturbance and the controller settings. The ideal PID controller is simply represented in Eq. (1):
where m is the variable manipulated by the controller, and P, I and D are its proportional band in percent and integral and derivative time settings respectively. This formula may be evaluated both before and after an upset, when the deviation e and its time derivative are both zero. If the controller output has changed between those two steady states, there will be an integrated error:
Equation (3) applies equally to interacting and non-interacting PID controllers and PI controllers as well. There are some obvious conclusions to be drawn from it: To minimize the cost associated with IE, minimize the feedback control effort Δm required to respond to an upset (by using feedforward), and reduce the P and I settings (without sacrificing stability).
Unsafe-Side Variations
When the controlled variable wanders to the unsafe side of the specification limit, decisions have to be made. In failing to meet requirements upon which the product is to be sold, it must be set aside for blending with better-than-acceptable product, rejected to a less-valuable use or rerun. All of these operations are costly and cause disruptions affecting production rate. Operators avoid them if at all possible.
The alternative is to adjust the setpoint of the composition controller so that it exceeds the specification limit by the expected variations in quality under all but the worst cases. This is also costly in that it results in quality giveaway all the time. Yet this is the normal condition of most product-quality controllers in most plants, and offers the largest economic potential for tighter control in processing plants universally. To minimize giveaway in the face of unsafe-side variations, the amplitude of those variations must be minimized.
The curves in Fig. 1 are typical of a loop responding to a step change in load—the flow or composition of one of the ingredients entering the process—and the subsequent reaction of a well-tuned PID controller to that upset. A step was selected as it is the most difficult disturbance for a control loop, containing all the frequencies from zero to infinity—a step has the same power spectrum as white noise. At the zero-frequency end, it requires a permanent change in controller output, necessitating integration. At the other end, its rise time is zero, requiring vigorous proportional and derivative action. Finally, it is commonly encountered when equipment trips or is suddenly switched, and is the easiest test to administer. In their monumental work on controller tuning in 1941, Ziegler and Nichols used a pneumatic bias regulator in the controller output to simulate a load change at the process input. We can do it by transferring the controller to Manual while in a steady state, stepping its output and immediately transferring to Auto.
The well-damped step-load response curve has a first peak much higher than all the rest. If that can be cut down, the setpoint can be moved closer to the specification limit. Since it is a single peak, it is easily attenuated by any downstream capacity, allowing the margin between setpoint and specification limit to be biased somewhat less than the expected peak deviation. The amplitude ratio Ar of output to input for a cycle passing through downstream capacity of time constant Τ1 is
where Τo is the period of the wave. Where the time constant of the downstream capacity is long compared to the period, the operating margin can therefore be small. For example, a 1-minute cycle passing through a 10-minute capacity is attenuated by a factor of 62.8. With very large capacity, load upsets are equally likely in both directions, and IE over time can approach zero.
Peak deviation and period are then the key factors affecting the operating cost for unsafe-side variations. The first step in minimizing peak variation and period following a load change is to be sure that the controller is paired with the manipulated variable having the most influence over it, and less over other associated variables. (This concept was presented in Ref.1 with regard to distillation and will not be repeated here.) Having made that pairing, performance is then determined by controller selection and tuning.
Minimum-IAE Tuning
As useful as IE is in evaluating loop performance, it is incomplete. IE can be lowered by successive reductions in proportional band and integral time according to Eq. (3), but that also reduces the damping of the loop, leading to instability. A more complete performance criterion is Integrated Absolute Error (IAE), which has a minimum value that can be reached by proper tuning. The sign of the deviation e is simply removed before integration. In this way, the IAE of an oscillating loop will increase without end—IAE penalizes both load response and instability, reconciling them. For the curve of Fig. 2, IAE is only 9% higher than IE, so IE is being effectively minimized by minimizing IAE.
(Other related criteria are Integrated Square Error (ISE), where the deviation is squared before integrating, also eliminating the sign, and the Integral of Time and Absolute Error (ITAE) that penalizes errors increasing with their duration. The square function has no economic significance, but is simply mathematically convenient, and the time function of ITAE is not applicable to continuous processes.)
Fig. 2. The minimum-IAE curve is identified by its decay ratio and overshoot.
There can be difficulties in calculating IAE in the field; for example, any noise will cause it to increase without limit. However, it does not need to be monitored to achieve minimum-IAE tuning, because its closed-loop load-step response curves have characteristics readily identified in simulations that can be duplicated in the field. After having simulated many of these loops, the characteristics shown in Fig. 2 are found to be common to most: They are low damping ratio δ, small overshoot Ω, and short period Τo. Fig. 2 was obtained by stepping the load to a distributed lag under PID control with these results:
The time scale is normalized by dividing by Στ, the sum of all the lags in the distributed process—it is identified by the time required for 63.2% complete response from the initiation of a step input in the open-loop. (See Ref.2 for more information on this process common to heat exchangers, multistage columns and stirred tanks.) Essentially the same values of decay ratio and overshoot represent minimum-IAE response for other processes using other controllers; the period varies with the process dynamics and the type of controller.
There are many different methods used to tune controllers, with a wide range of effectiveness. Some are intended to optimize setpoint response, but these are not recommended, as they invariably compromise load response. Once a controller has been optimized for load response, setpoint filtering can then be added where necessary. The economically important loops in a process plant—controlling composition, temperature and pressure—operate at constant setpoint all the time, but are regularly exposed to load variations.
Fig. 3. Increasing proportional band and integral time increases IE.
Most tuning methods result in sluggish load response, costly in IE, peak deviation, and period. Some methods set integral time equal to the primary process time constant, whereas minimum-IAE requires it to be half that value for a PI controller and one-fourth for a PID controller. Two examples are compared in Fig. 3 against a minimum-IAE curve. Doubling the proportional band increases peak deviation by 44% as it doubles IE—heavier damping bought at a high price. Doubling the integral time has little effect on peak height as it doubles IE—it principally affects overshoot. These two responses can guide the user as to which parameter needs adjusting.
A common operating concern is robustness, that is, to remain well away from stability limits. With minimum-IAE tuning of the PID controller, the gain Kp of this process can increase by 80% before instability is reached, and Στ can increase by 75%—very acceptable margins both. If the process parameters are more variable than that, compensation should be applied, either through valve characterization or adapting the controller settings as a function of flow, needed when controlling heat exchangers2.
Another objection to tight tuning is measurement noise, which the proportional and derivative gains can pass along to the valve, causing excessive wear. Rather than detuning the controller, filtering may be applied, with the caution to use as little as necessary, for it augments the IE function:
where Τf is the filter time constant, and Δt is the sampling interval of the controller. In addition, the filter time adds to the dead time in the loop, requiring a further increase in all three controller settings. Sampling has a similar effect.
Controller Selection
Fig. 4 gives the step-load curves for three controllers, all tuned for minimum-IAE response. Derivative action is very effective against the multiple lags of distributed processes, as demonstrated in the substantial improvement of PID over PI control. Derivative is under-utilized in industry for a variety of reasons, but should be used whenever possible in high-value composition and temperature loops, for its obvious advantages in reducing peak deviation, IE and period.
Further reductions in those same curve features can be achieved by adding dead-time compensation τ to the PID controller, a capability that has been available for about twenty years, but rarely used. The controller dead time essentially delays integration by an amount similar to the process dead time, allowing a much shorter integral time. The IE formula for this controller is the same as Eq. (6), with controller dead time taking the place of Δt. Optimum settings for all three controllers on a distributed process are given in Table 1, along with the resulting IE, peak deviation e1, and period of the loop Τo, all normalized by division by process gain Kp, time constant Στ, and disturbance size expressed in terms of equivalent change in controller output Δm.
Table 1. Optimum controller settings and resulting response characteristics
The PIDτ controller has the highest performance available in a commercial product, but is rarely used owing to its robustness limitations. It is difficult to tune, because a mismatch between its dead time and that of the process in either direction can cause instability. Beginning from a condition of optimum tuning, limits of stability are reached when Στ increases by 37% or decreases by 8.5%, and when process gain increases by 25%. It has been successfully applied to controlling steam temperature from a superheater by programming all four settings as functions of steam flow3.
Feedforward Control Structure
If feedback alone cannot satisfy the economic objective, feedforward will be able to, but only if properly engineered. Figure 5 illustrates three methods of applying feedforward to control composition or temperature, two of which have serious limitations. In the top illustration, the process load—the feed rate to the process, for example—is added as an impulse function to the output of the PID controller. The impulse function is the difference between the measured value of the load and its lagged value; this is then multiplied by an adjustable gain K, and added to the PID output to set the manipulated flow. The gain adjustment is used to calibrate the system, so that a sudden change in load will produce the correct change in the manipulated flow that will cancel its effect on the controlled variable.
In the steady state, there is no difference between the load and its lagged value. This is considered operationally desirable, in that the output of the controller and the manipulated flow have the same steady-state value, as in a conventional cascade system.
However, it has a profound defect: After a load change has passed, the feedforward compensation gradually returns to zero, requiring the PID controller to move its output to the new steady-state value dictated by the load. In essence, feedforward correction is only dynamic—there will be a Δm required by the controller, which will have to integrate an error to get there. The IE is, therefore, no different with or without impulse feedforward. The impulse acts to flatten the disturbance, with the result that the same IE is spread out over a longer time. Peak deviation alone is reduced—but there is a better way.
Fig. 5. Three possible methods of applying feedforward control.
In a linear feedforward system, the load variable passes through a dynamic compensator (if required) and then is added to the controller output with a gain adjustment as shown. Again, the gain is set so that the effect of the load change is canceled by the resulting change in setpoint of the manipulated flow. This is the preferred arrangement when the process is linear and its gains are constant.
The best example of its application is that of boiler drum-level control. The level responds equally to the load—steam flow—and the manipulated flow of feedwater. The feedforward gain is thereby constant at 1.0. Linear feedforward control of boiler drum level has been applied as early as 1929, in the "three-element" feedwater system.
In the control of composition or temperature, however, the gain of the controlled variable to load flow is not constant. It varies with both the feed condition and the product composition or temperature setpoint. Feedforward gain can be set to match the process gain initially, but it will be incorrect when feed conditions or product specifications change. For example, the ratio of high-octane blending ingredient to the flow of the main stock varies with the octane rating of that stock and the setpoint of the octane controller.
We see linear feedforward used widely in matrix-based multivariable systems. The process is first tested extensively to estimate all the gains relating load, manipulated and controlled variables, both steady-state and dynamically. The gains are then entered into the control matrix and fine-tuned for performance. But since the process gains change with feed conditions and product slates, after a while they may no longer match the feedforward gains set into the matrix, so control deteriorates—IE following load changes is no longer zero. Accounts of lost performance over time are very common with installed linear multivariable systems.
Composition and temperature measurements are properties of a flowing stream. The heat content of a flowing stream is the product of its flow and its temperature rise above inlet conditions. Similarly, the rate of high-octane blending ingredient needed to reach the blend specification is the product of the main stock flow and the rise in octane number required. Multiplication is a bilinear operation—the output of a multiplier is linear with both of the inputs. Multiplication of variables is an essential function in feedforward control of composition and temperature. Accurate multipliers only became available in pneumatic and electronic instrumentation in 1960, which is when feedforward control began to be applied to heaters and distillation columns.
The bottom system in Fig. 5 multiplies the load variable—feed flow—by the PID output. A very common example of a bilinear feedforward system is the control of stack-gas oxygen by manipulating the air-to-fuel ratio in a combustor. The oxygen-controller output then represents the ratio of the manipulated airflow to the load fuel flow. This ratio is not a constant, but varies with both the heating value of the fuel and the setpoint of the controller in terms of percent oxygen in the stack gas. This setpoint is varied with load in most boilers, and the heating value of the fuel varies widely in mixed-fuel installations. When either of these parameters changes, the oxygen measurement is upset, and the controller must readjust its output to restore a balance. In doing so, it is not only returning the controlled variable to setpoint, it is also recalibrating the feedforward gain to match the new process gain.
The multiplier also serves another purpose: gain-compensating the feedback loop. The gain of a bilinear process varies inversely with flow. For example, a step in the flow of octane enhancer to a blender will have twice the effect on the blend octane at half the production rate as it does at full production. Likewise, a step in steam flow to a heat exchanger will have twice the effect on the temperature of liquid leaving at half flow than it does at full flow. This has led engineers over the years to use equal-percentage valves for temperature control, as the valve gain varies directly with flow delivered. In the bottom diagram of Fig. 5, the feedback-loop gain from the controller passes through the multiplier, whose gain varies with the load variable, the flow through the process.
Applying Dynamic Compensation
Some processes have their load and manipulated variables entering at different points physically, with different dynamic effects. A case in point is the heat exchanger, where liquid exit temperature responds faster to the flow of liquid entering the tube bundle than to steam flow entering the shell. Stepping the manipulated steam flow down in response to a drop in liquid flow will then result in a temporary rise in exit temperature until a new steady state is reached. Figure 6 shows the solid blue curve as the result of a step down in liquid flow to a simulated heat exchanger using static feedforward alone—no dynamic compensation or feedback. While the peak deviation is less than the black curve under PID control, it has a pronounced integrated error owing to the dynamic imbalance in the process.
Static feedforward that is inaccurate results in offset if there is no feedback controller to correct the error brought about by a gain mismatch in the forward calculation. Static feedforward that is accurate provides all the change in the manipulated variable required to match the new load, so that the controlled variable will return to setpoint without any effort on the part of the feedback controller. The controller output is therefore the same before and after the load change. The PID controller was left in Manual to produce the solid blue curve; then the load change was repeated with the controller in Auto, producing the dashed blue curve. The controller made a correction in an attempt to reduce the deviation from setpoint, though too late to have much effect on its peak value, and then had to remove the correction altogether approaching the new steady state. The result is an IE of zero.
Achieving a dynamic balance requires matching the dominant lag in the load path with an equal lag in the path of the manipulated variable, and canceling the lag in the manipulated-variable path with an equal lead. The dynamic compensator commonly used in feedforward control is, therefore, a lead-lag function, sometimes with a little dead time added where necessary. In the case of the heat exchanger, the lead time constant needs to be greater than the lag, giving a dynamic gain—their ratio—typically in the range of 1.5-3.
The IE in the response curve will be zero without feedback when the difference between lead and lag time constants of the compensator matches the difference between the two process lags. This is a useful guide to tuning the compensator, but is insufficient to achieve a dynamic balance—the ratio of lead to lag must also match that of the process lags. If the peak deviation develops on the side driven by the load, the ratio of lead to lag is not high enough. Reducing both lead and lag settings while keeping their difference constant will increase their ratio while keeping IE at zero. The red response curve is typical of what can be achieved by careful tuning—a minimal peak deviation and an IE of zero.
Once-through processes such as heat exchangers without recirculation have an additional complication: their dynamics vary inversely with flow. Fixed values of lead and lag can then be correct at only a single flow rate. As flow increases, both process lags decrease, and so does their difference. As a consequence, IE can be zero at only one flow. This is easily correctible, however, by programming the lead and lag settings to vary inversely with measured flow. The integral and derivative settings of the PID controller should also be programmed in the same way. This was done in the simulation results appearing in Fig. 6.
References
- Shinskey, F. G., "Multivariable Control of Distillation,"Control, May-July, 2009. (see Multivariable Control of Distillation,
Multivariable Control of Distillation, Part 2, Genie in a Bottle: Distilling Greg Shinskey into Software - Shinskey, F. G., "Controlling Distributed Processes,"Control, May 2007.
- Shinskey, F. G., "PID-deadtime Control of Distributed Processes, Control Engineering Practice, 9 (2001) 1177-1183.
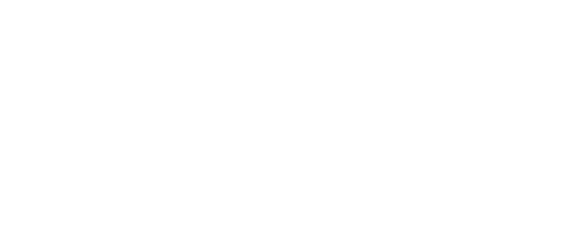
Leaders relevant to this article: