In the early 2000s, process manufacturers rushed to hire cohorts of six sigma black belts. These continuous improvement experts were placed in process plants with directives to maximize throughput and minimize defects using a host of statistical analysis techniques, with SQC and SPC among the most popular. Fast forward two decades, and a similar hiring frenzy has emerged, but this time the focus isn't on the color of one’s belt, but instead on the depth of an employee’s data science and analytics capabilities.
As process manufacturers catch the data science wave, they face an early decision of whether to upskill or onboard. There are pros and cons to each strategy.
Looking outside the organization
The phrase "build it or buy it" isn’t often associated with staffing, but it fits nicely when talking about creating new organizational capabilities. In this case, let’s take “buy it” to mean the intentional hiring of people with the skills required to close the data science skills gap.
There are certainly advantages to building a corporate manufacturing data science team by hiring the best and brightest data scientists. They’ll have a strong foundation in the mathematical principles that power machine learning programming libraries. They’ll also know the right algorithms to apply for the right use cases, and they’ll be fast and efficient at wrangling data into the right format for applying said algorithms. They’ve been programming for years and can bounce back and forth between the various languages required by existing data science software stacks in process manufacturing companies.
Now, for the challenges of "buy it," let’s begin with budget. When manufacturing companies look to hire data scientists, they must understand their competition. Large energy company A doesn’t just have to beat large energy company B. When recruiting data scientists, process manufacturers must be competitive with big tech, investment banking and consulting firms. This makes hiring a cohort of data scientists much more costly than the “build it" approach.
Hiring data scientists will build immediate competency in this area, but these experts will fail if they’re working in a vacuum because they’ll need support from subject matter experts. So, the next challenge faced by process manufacturers taking the "buy it" approach is connecting data scientists to the manufacturing processes they’re supporting.
Just like the models they create, data scientists must be continually trained and retrained in how the results they generate relate to the process to avoid false positives and meaningless correlations. A data scientist telling you that your control system is working as designed—unhelpful! A data scientist providing early detection of a critical equipment failure—helpful!
To upskill or not to upskill?
The "build it" approach involves leveraging training and technology to build data science capabilities into a process manufacturer’s existing workforce.
When evaluating the feasibility of upskilling an existing labor force in data science and analytics technologies, it's important to understand the starting point of foundational knowledge. Data science curricula are becoming more common offerings in university engineering programs, which means new entrants to the workforce are likely to have some understanding of statistics and analytics.
For the existing engineering workforce, particularly those who've been out of school for more than five years, it's quite possible that they may have never taken a statistics course. In a slightly biased poll of 25 classically educated chemical, mechanical and electrical engineers now working in the data science and analytics industry, 35% of respondents reported that there was no statistics requirement in their undergraduate coursework.
Creating a training program for a workforce with a range of baseline analytics understanding and programming capabilities is extremely challenging. Therefore, providing multiple starting points for different personas with varying experience levels is important to ensure employee engagement and adoption of new data science and analytics practices.
Another key is offering different training options for different styles of learners. People may learn by reading, seeing, speaking or using or any combination of styles. It would be unrealistic to assume that one training format will be effective for a diverse manufacturing workforce.
Another challenge in effective upskilling of current employees is identifying the right tools that reduce the activation energy of learning. Requiring an entire engineering workforce to learn Python programming is unrealistic. Requiring everyone to become Excel power users is outdated. Transforming an existing workforce into an army of citizen data scientists is best achieved through technology investment in self-service analytics software offering different experiences catering to the different user personas in a manufacturing organization.
The good news? These people are experts in your manufacturing processes! They have years of experience working with the data, and know what to immediately throw out, what to ignore, and what might be interesting. They may not understand the mathematics fundamentals of the machine learning algorithm they’re working with, but they can apply them and interpret the results quickly, providing recommendations for operations teams to act on.
A hybrid approach
With pros and cons to both strategies for bridging organizational data science gaps, there's a middle ground that embodies the best of both. The most successful data science initiatives have adopted a hybrid approach of investing in degreed data scientists and building citizen data scientists by training.
Ensuring the success of a hybrid approach requires a commitment to removing organizational barriers to data and knowledge sharing. Cloud-based analytics applications are removing the IT hurdle of working only on systems within the site firewall. Knowledge capture and collaboration features help engineers document workflows, thought processes and assumptions, so downstream users of analyses can pick up where the last person left off.
Newly hired data scientists will be most effective when they work in collaboration with site manufacturing engineers and domain experts. This way, data scientists aren’t starting from scratch, but are instead building on each engineer’s domain expertise applied in data cleansing to remove abnormal operations and outliers, targeting events, and paring down the analysis to signals with process relevance. When a data scientist begins an analysis with an aligned, cleansed and contextualized data set from a process expert, the 80/20 rule of data science is flipped upside down (Figure 1).
Building out data science capabilities of any kind within traditional process manufacturing organizations will take time. Most process manufacturers today have about one data scientist for every 10-50 engineers. This imbalance makes a strong argument for the need to upskill existing workforces, whether or not the creation of a data science team is underway. Upskilled engineering resources working with self-service analytics tools can drive most use cases 90% of the way to completion, but collaboration with a data scientist can be integral to pushing an analysis over the finish line.
Allison Buenemann is an Industry Principal at Seeq Corp. She has a process engineering background with a B.S. in chemical engineering from Purdue University and an MBA from Louisiana State University. Allison has nearly a decade of experience working for and with bulk and specialty chemical manufacturers like ExxonMobil Chemical and Eastman to solve high-value business problems leveraging time series data. In her current role, she enjoys monitoring the rapidly changing trends surrounding digital transformation in the chemical industry and translating them into product requirements for Seeq.
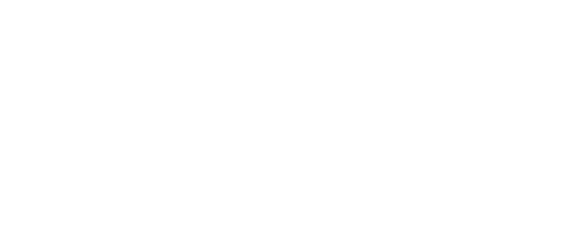
Leaders relevant to this article: