How IIoT can aid assets for CapEx and OpEx savings
"I come from Schneider Electric's software business focusing on asset management, and while this isn't process control, it does affect reliability," said Justin Thomas, business development manager at Schneider Electric. "Sensors and their data in historians and other components that can be enabled by IIoT are already out there, and all of this existing technology can be leveraged to increase reliability, reduce maintenance costs, and improve profits."
Thomas presented "Enterprise Asset Performance Management: Harnessing the Power of IoT for OPEX and CAPEX Savings" as part of the Downstream Oil & Gas Track on the first day of Schneider Electric's CONNECT 2016 Automation Conference this week in New Orleans.
"There are big benefits to the predictive analytics, including overall savings of 1-2% in operations costs.â Justin Thomas, business development manager, Schneider Electric spoke at Schneider Electric's CONNECT 2016 Automation Conference this week in New Orleans.
"Traditionally, preventive maintenance has been done on time-based schedules, and though this has gotten better with more timely data and performance reports, truly predictive maintenance has remained limited," said Thomas. "Though most users have some kind of asset management system, many aren't doing this kind of technical analysis yet, even though they want better data for maintaining their assets. Studies by ARC Advisory Group find that only 18% of asset failures are age-related, while 82% are due to random failures. This means preventive maintenance provides a benefit for just 18% of assets, while monitoring for predictive maintenance is recommended for the rest."Schneider Electric is the only provider of a fully integrated, end-to-end, comprehensive Enterprise Asset Performance Management (APM) solution that goes from sensing to visualization to recording to tracking, and plugs in models based on what equipment users already have in place. This enables our customers to exceed safety, reliability and performance goals through data collection, storage and analysis, which is coupled with actions and optimization for proactive maintenance execution. The result is improved workforce efficiency and decision-making; increased asset reliability and availability; and maximized economic return on industrial assets."
Thomas adds that users can also migrate to a more condition-based approach for maintenance by using Schneider Electric's Avantis Condition Manager software and/or its Avantis PRiSM Predictive Asset Analytics software with machine learning functions.
"Avantis Condition Manager automates the maintenance process by monitoring user-defined rules that initiate necessary maintenance activities," explained Thomas. "When conditions are known and definable, it uses rule-based logic. Maintenance rules are fixed and don't significantly change based on equipment loading, ambient or operational conditions. Avantis Condition Monitor also intelligently integrates plant process data with EAM systems. Typical users are maintenance managers, who needs a condition monitors (rules engine) to feed into their work-order management system.
"Meanwhile, Avantis PRiSM performs software-based modeling of equipment using advanced pattern recognition, and uses historical data to describe how a piece of equipment normally operates to build a model. Next, it continuously monitors behavior in real time, and provides alerts when the operation differs from the historical norm. It also delivers early-warning detection of equipment problems, as well as advanced analysis capabilities, including problem identification and root-cause analyses. Using current data, it also examines how all parameters of a system work together to affect asset performance, which allows users to analyze combinations of related events."
Thomas added that Avantis PRiSM core tools include:
- Early-warning event detection and management (alarm manager), which detects any changes in existing assets, identifies anomalies, and delivers alerts about them.
- Trend analysis that indicates what causes are contributing to the anomaly.
- Diagnostics advisor (fault diagnostics) that identifies likely causes of anomalies, indicates how far they are from normal performance, and shows their likely sources, including a confidence percentage.
- Case management that documents and tracks anomalies, then stores them in a library. Tracking is also possible using financial metrics.
- Reporting to allow distribution of information about anomalies and other issues across all of a user's staff and components. This tool also has a dialog box that allows all team members to see what's happening, and discuss what to do about it.
"Most users only look at alarms, but they don't see these situations develop," added Thomas. "When users add predictive analytics, they can see the beginnings of deviations, and address formerly unplanned outages before they can occur."
For example, a company recently started one of its units after an outage; used Avantis PRiSM to find a vibration step-change on a LP turbine; notified its engineers and plant; collected vibration data and retired the unit for inspection; and discovered broken bolts on the lower half of the flow sleeve that were causing the sleeve to contact the L-O blades. "The upper half of the flow sleeve was no longer supported by lower half, and so finding this avoided damage to multiple stages of the blades, packing and diaphragms," added Thomas. "This avoided initial costs of $4.1 million, and total avoided costs estimated at $18 million during 2015."
Likewise, at Southern Co., PRiSM indicated an increase in vibration on multiple bearings. Its staff found a BFP coupling shim pack that was on the verge of failure, which saved about $260,000 immediately, and resulted in an estimated $4.5 million in avoided costs in 2015.
"PRiSM also reduces unexpected maintenance, maintains data quality reliability, and enables web alarm management," says Thomas. "There are big benefits to the predictive analytics, including overall savings of 1-2% in operations costs. If you have unplanned outages, there's a reason for them, but there's another way to reduce them by taking advantage of data you already have. These tools can achieve catches from $10,000 to $50 million, and just imagine if you were on the team that finds them." Â
Latest from Data Acquisition
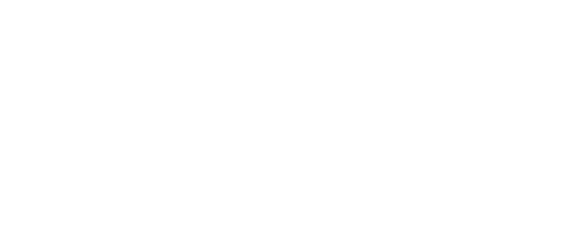
Leaders relevant to this article: