From the Editors of CONTROL
Asset Management Excellence
We are trying to maximize the lifecycle and the long term asset value of the large equipment assets. We are trying to optimize our maintenance. At a strategic level we’re talking about refurbishment and replacement of aging assets. With aging assets it is all about when you do that. We have tools to help us understand the plant equipment condition.
You can replace before failure. This is a low risk option, but it is very expensive. The strength of the Predictive Asset Management system is that it works for all levels of equipment from large to small assets.
You can replace after failure. This, too, is a suboptimal choice. Emergency replacement can cost as much as five times normal purchase cycle cost, not to mention the potential environmental damage. “We have transformers in a World Heritage site,” Gregory said, “and chucking 15,000 liters of transformer oil down the ridge just isn’t right.”
Plant condition monitoring: “you must understand the condition of your asset or you’re just a victim waiting for an accident to happen.” But if you do understand conditions, you can make informed decisions about maintenance and repair.
The problem with our first condition monitoring system was that we were flooded with data. “The problem with data is that you have to finally get people to look at it. We just didn’t think it was good enough to get someone somewhere to spend time looking at the data.”
We thought we needed a Plant Asset Management System. What is different about a PAMS is that it is designed to do something with the data from the condition monitors, not just collect the data.
We believed that we needed a predictive PAMS, and we found in 2003 that there was absolutely no such system on the market. We specified what we wanted, and found that Matrikon was successful in obtaining the project. “There was actually daylight between Matrikon and the next best company. And Matrikon was the only one of the six companies on the short list I’d never heard of.”
We had 10 years of CMMS data from Maximo, 3 years of PI data, and lots of process data. What we didn’t have was the bit that pulled it all together. That’s the PAMS.
The PAMS analyzes the data from Maximo and the PI database, and notifies the right people of the results of the analysis. Then somebody can actually do something about the data.
Gregory showed asset overview slides that gave overall health values for critical assets like transformers and turbines. “We needed an icon for ‘governors’ so we picked a photo of the Governator, since the PAMS identifies bad actors as well, eh.”
Meridian uses the PAM to predict things like “days to transformer dry out” and other Key Maintenance Indicators. Managers can access maintenance summary screens that allow drill-down into Maximo to see work order status. They can also see alert summaries, so they can keep track of the “hot” items they’re responsible for. The PAM can email reports to key people so they can be reminded rather than expecting them to find the right screen every day.
Gregory showed the “custom dashboard builder” and called it one of his favorite pieces of the software. Plant managers and engineers can build their own dashboards to keep track of assets they are worried about.
We don’t expect this system to warn us of catastrophe everyday. What we expect is an early warning system. The system is like the barrier at the top of the cliff that keeps you from going off. It is the light on the dashboard that tells you that something is coming and you need to do something about it.
In the future, we expect to have twice as many assets in the model by 2009.
Best Practices: How to Stop Drowning in a Sea of Alarms
This morning’s final session in the “Best Practices” track focused on alarm management. Dr. Joseph Alford, recently retired from Eli Lilly, and Mik Marvan, Matrikon’s alarm management product manager, threw a life preserver to automation professionals drowning in a tsunami of alarms. Dr. Alford condensed 35 years of batch processing alarm management experience into a few key slides.
To rescue the average batch processing plant floor operator from the more than 3,000 alarms he has to account for on a given shift, do the following things, says Dr. Alford
Using the “alarm management” system on a car as an example, he demonstrated the characteristics of an ideal alarming system. In a car, the “alarms,” such as a low fuel gauge, represent an “abnormal” situation that requires a response. The alarm systems are accurate and reliable. Ordinarily people don’t question whether the low fuel indicator really means they have to stop for gas. These alarms permit a reasonable time for response: The driver has time to get to the gas station. Most important, the alarms are few in number, in spite of the fact that cars are complex systems. Why can’t the alarm systems in our batch processing operations have the same characteristics, asks Dr. Alford.
Allowing for the additional characteristics of batch operations, such as multiple process steps, multiple phases, process loads, set points that are a function of time, and few, if any steady-state operations, these are his recommendations for building such a system.
- Adhere to the basic definition of alarms—“abnormal situations requiring a response.” Just doing this will knock out about 75% of alarms, says Alford.
- Tag alarm records with sufficient information needed to use the information for displays, sorts, queries and report generation. Include lot numbers, category, priority, process step/phase, etc.
- Generate more “intelligent” alarms that make use of all available relevant information, such as those that include if-then rules incorporating redundant sensors, trend slopes, and other correlated variables.
- Eliminate nuisance alarms. Do not include “notifications” on alarm displays. Make every effort to separate information messages from alarms.
- When color-coding displays, use each color only one time. If red indicates a high-priority alarm, don’t use that color for anything else.
- Minimize multiple alarms for each event.
- Ensure that alarms alert, inform and guide. They should tell the operator everything he needs to know about the alarm. “Most commercial systems do a great job of alerting, a mediocre job of informing and a bad job of guiding,” says Alford.
- Set up a system that allows sufficient mining of alarm data. Make records easy to access and understand. Include sufficient alarm tag information. Include “relative time” information. The fact that the alarm occurred at 3:00 a.m. is less important than that it occurred 2 hours into, say, a sterilization process. Include utilities that allow the creation of pareto charts and other trending information and those that include the ability to combine discrete and continuous trends. This is all about providing the necessary context.
Mik Marvan concluded the session with showing how Matrikon’s Alarm Management System can help companies implement these best practices. See www.matrikon.com for detailed information about this system.
Operational Excellence: Tai-Ji Steps Out at Hovensa Refinery
Step testing usually requires a lot of steps. As a result, traditional step tests and model identifications are very time consuming. Users must step a variable, wait for steady state, step it again, wait again, step, wait, step, wait, etc. This process also is costly and intrusive to process operations, and can require six to eight weeks or more of plant engineers’ time for new or maintenance projects. And, these days, there often aren’t enough experienced staffers to do these jobs anyway.
So, wouldn’t it be nice to find a way to automatically dance through step tests and modeling procedures? You bet.
That’s just what Bob Tkatch, process engineer at Hovensa LLC’s application engineering department, and his colleagues thought when they evaluated and adopted Tai-Ji on-line, multi-variable identification software and Matrikon’s Control Performance Monitor control performance monitor at the St. Croix, U.S. Virgin Islands-based refinery’s Crude Unit 6 MPC application with 34 multi-variable (MV) controllers and 90 CVs covering two furnaces, an atmospheric tower, and a naphtha stabilizer.
During the plant test, an existing MPC controller is online and active in stabilizing the operation, while the test program moves all MVs simultaneously. It took only eight days to conduct the closed-loop plant test, and perform model identification and review. The new plant test was found to be non-intrusive to the plant’s normal operations allowing the operators to concentrate on their normal duties. The refinery’s latest multi-variable predictive controller (MPC) retesting was completed automatically by stepping multiple independent variables simultaneously, while the MPC controller was in service.
Hovensa is a joint venture between a subsidiary of Amerada Hess and a subsidiary of Petroleos de Venezuela, S.A. (PDVSA). The refinery can process approximately 500,000 barrels per day (BPD) of crude oil, which makes it one of the largest such facilities in the world. Hovensa includes four crude units, three vacuum units, eight DDs, three platformers, four sulphur recovery units, four amine units, 198 large tanks, and other facilities.
Tkatch reported that automated testing was especially welcome at Hovensa because they only have two APC engineers, or four during maximum staffing. Also, he says automated testing was needed because many MV controllers begin to perform poorly over time due to model degradation or inaccuracies, and so their models no longer reflect operating conditions. This degradation can be caused by long unit run-time, fouling exchangers and valves, changing operational objectives, and/or unit damage.
“A well-tuned, well-maintained MV controller pushes multi-variable constraints more effectively, providing opportunities for more efficient and profitable operations,” says Tkatch. “With Tai-Ji, MPC plant test projects and modeling projects can be done with limited staff, budget, time, and intrusion into the daily life of operations. We use Tai-Ji because it saves time and it works. Using Tai-Ji takes away the misery of doing traditional step testing.”
To accomplish these goals, Tai-Ji automates closed-loop and open-loop testing by first stepping variables automatically within defined limits. All defined variables then are stepped simultaneously, which enables Tai-Ji to provide far more steps per variable in its step test than a traditional step test could ever accomplish in the same time period. “Stepping all variables simultaneously provide far more steps per variable in about 25-30% of the time required to do a traditional test because you’re not stepping and waiting, stepping and waiting,” says Tkatch. “We can start the automated test, go do our other work, and just check on the test once in a while.”
Some of Tai-Ji’s main features include:
- Automatic test signal design and generation
- Automatic step testing—implementation of the steps to the process—in a closed-loop or open-loop mode
- Real-time monitoring of steps, variable limits and current process operation
- Real-time adjustment of variables stepped, their step sizes and Tai-Ji limits
- Model identification with model quality analysis providing immediate feedback as to test step performance and data quality
Meanwhile, Process Doctor prepares the application for its step test by surveying regulatory controller performance, and identifying bad actors, such as controllers that aren’t performing well, and helping to determine if they need tuning, maintenance, or repair. Once the regulatory controllers are working well, the step test is ready to begin.
Tkatch says users should ensure that their data is being archived in at least two different places, set up the Tai-Ji modeling analysis using common sense combinations of variables, and keep the model analysis setup simple, but inclusive. Then, after approximately 24 hours of stepping, models can be run. Model results are graded using estimated error bounds, including:
- A = very good, high quality model
- B = good (collecting more data may improve this model)
- C = marginal (Larger steps will generally improve this model)
- D = poor model, or no model (additional data collection required)
Tkatch adds that model identification is successful if most expected models have A and B grades, but this doesn’t not mean that all models must be A or B. Next, he advises users to:
- Look at step response plots, and use a scaled-response option to identify dominant models versus less dominant models when evaluating model qualities.
- Use frequency response to judge the spread of model errors. Low frequencies need to be accurate.
- Use model simulation plots to assess predictions. Error of less than 30% is adequate in practice.
- Use slicing if CVs are saturating or there are calibration periods in the data set, or huge unmeasured disturbances.
- For existing transforms, ensure that these transforms are applied before sending data to the model block.
- Then, if an MV has consistently poor models, focus on increasing the step sizes or frequency content.
- Once satisfied with models for some of the variables, remove them from the step test simply by un-checking the select box in the web interface. These variables will not be stepped any longer, but the rest of the test continues.
So far, Hovensa has now used Tai-Ji for stepping and model identification on five process units. These include revamping its Crude Unit 6 in 2005 with two sub-controllers on its crude tower and stabilizer; revamping its fluid catalytic cracking (FCC) unit in 2006 with three controllers; and revamping its BTX unit and distillate desulphurization unit in 2007. Tkatch adds that Hovensa plans to conducted closed loop tests on its Crude Unit 5 and Vacuum Unit 3 this year.
Continue Reading
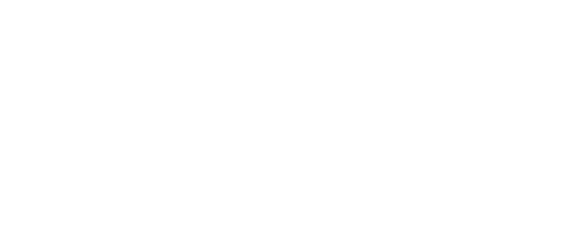
Leaders relevant to this article: